Frobenius Norm and Low Rank Approaximation
Contents
Frobenius Norm and Low Rank Approaximation¶
1.Brief Singular Value Decomposition (SVD)¶
We first need to compute the SVD before going for low rank approximation. SVD is factorization of m x p matrix(A).
Where,
U and V is orthogonal matrix, where U is m\(\times\)p and V is p\(\times\)n matrix
S is singular diagonal of p x p matrix
\(S =\begin{pmatrix} \sigma_1 & 0 & \cdots & 0 \\ 0 & \sigma_2 & \cdots & 0 \\ \vdots & \vdots & \ddots & \vdots \\ 0 & 0 & \cdots & \sigma_p \end{pmatrix}\)
\(A = [U_1,U_2...,U_p] {\begin{pmatrix} \sigma_1 & 0 & \cdots & 0 \\ 0 & \sigma_2 & \cdots & 0 \\ \vdots & \vdots & \ddots & \vdots \\ 0 & 0 & \cdots & \sigma_p \end{pmatrix} } [V_1,V_2,...V_p] = \sum_{j=0}^p\sigma_jU_jV_j^T \)
The vector \({(U_j)}_{j=1}^p\) and \({(V_j)}_{j=1}^p\) are left and right singular vectors, forms othonormal of the range \(A\) and \(A^T\) respectively. where as \({(\sigma_j)}_{j=1}^p\) are the singular values of A. [Note - \(\sigma_1 \ge \sigma_2 \ge.....\ge \sigma_p \ge 0 \)]
2.Frobenius norm¶
Frobenius norm of matrix A is denoted as \(||A||_F\) , can be formulated as
\( A = (\sum_{ij}|A_{ij}|^2)^{1/2}\)
also \(∑||A_{*i}||_2^2 = ∑||A_{i*}||_2^2 \)For any row \(a_j\) of matrix \(A\)
\(||a_j||_2^2 == \sum_{i=1}^r(a_j^TV_i)\) , where \(V_i=i^{th}s-vector\)
\(||a_j||_2^2 = \sum_{j=1}^n \sum_{i=1}^r(a_j^TV_i)^2 = \sum_{i=1}^r|AV_i|_2^2 = \sum_{i=1}^r\sigma_i^2\)
therefore,
\(||A||_F^2 = \sum_{i=1}^r\sigma_i^2\)
3. Low rank approaximation¶
Let metrix \(A\in \mathbb{R}^{m{\times}n}\) with rank \(\le min(m,n)\). THe low rank approximation of \(A\) is to find another matrix B with rank-k [\(B_k\in\mathbb{R}^{m{\times}n}\)] which approximate \(A\) and has less rank than \(A\).
To find the best \(B_k\) , and how closely \(B_k\) approximates \(A\). we can use the concept of frobenius norm as followed
\(||A-B_k|| = min||A-B_k||_F: A\in \mathbb{R}^{m{\times}n},r(B_k) \le k\)
SVD based low-rank approximation -
we aware of following equation or term from SVD\(A = USV^T = \sum_{j=0}^p\sigma_jU_jV_j^T \)
The best-\(k\) approximation for matrix \(A\) is given by
\(A_k =\sum_{j=1}^k\sigma_jU_jV_j^T \) , where \(k \le rank(A)\) , where \(U_k = [U_1, U_2, ..., U_k] , V_k = [V_1, V_2, ..., V_k]\ and\ S_k = diag[\sigma_1, \sigma_2, ..., \sigma_k]\)
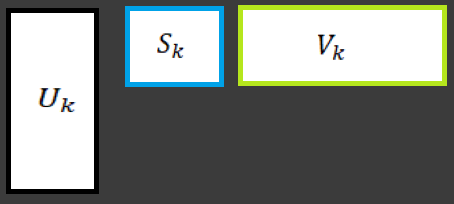
Fig. 35 Low rank approaximation¶
using frobenius norms
\(||A-A_k||_F=||A-B||_F\) , where \(rank(B)\le k\)
In terms of singular value,
\(||A-A_k||_F = (\sum_{j=k+1}^p\sigma_j^2)^{1/2}\)
simple example -
To find rank-2 approximation of matrix A
\(A = \begin{pmatrix} 3 & 2 & 1 \\ 0 & 2 & 1 \\ 0 & 0 & 1 \end{pmatrix}\)
Now
\(A = USV_T\)
\( = \begin{pmatrix}
0.91 & 0.42 & 0.02 \\
0.41 & -0.87 & -0.26 \\
0.09 & -0.24 & 0.97
\end{pmatrix} \times \begin{pmatrix}
4.04 & 0 & 0 \\
0 & 1.7 & 0 \\
0 & 0 & 0.87
\end{pmatrix} \times \begin{pmatrix}
0.67 & 0.73 & 0.08 \\
0.65 & -0.54 & -0.53 \\
0.35 & -0.41 & 0.84
\end{pmatrix}^T\)
\(A_2 = \begin{pmatrix} 0.91 & 0.42 \\ 0.41 & -0.87 \\ 0.09 & -0.24 \end{pmatrix} \times \begin{pmatrix} 4.04 & 0 \\ 0 & 1.7 \end{pmatrix} \times \begin{pmatrix} 0.67 & 0.73 \\ 0.65 & -0.54 \\ 0.35 & -0.41 \end{pmatrix}^T\)
\(A_2 = \begin{pmatrix} 2.99 & 2.01 & 0.98 \\ 0.02 & 1.88 & 1.1 \\ -0.07 & 0.45 & 0.29 \end{pmatrix}\)
The above matrix A is of rank 2, which is requirement.